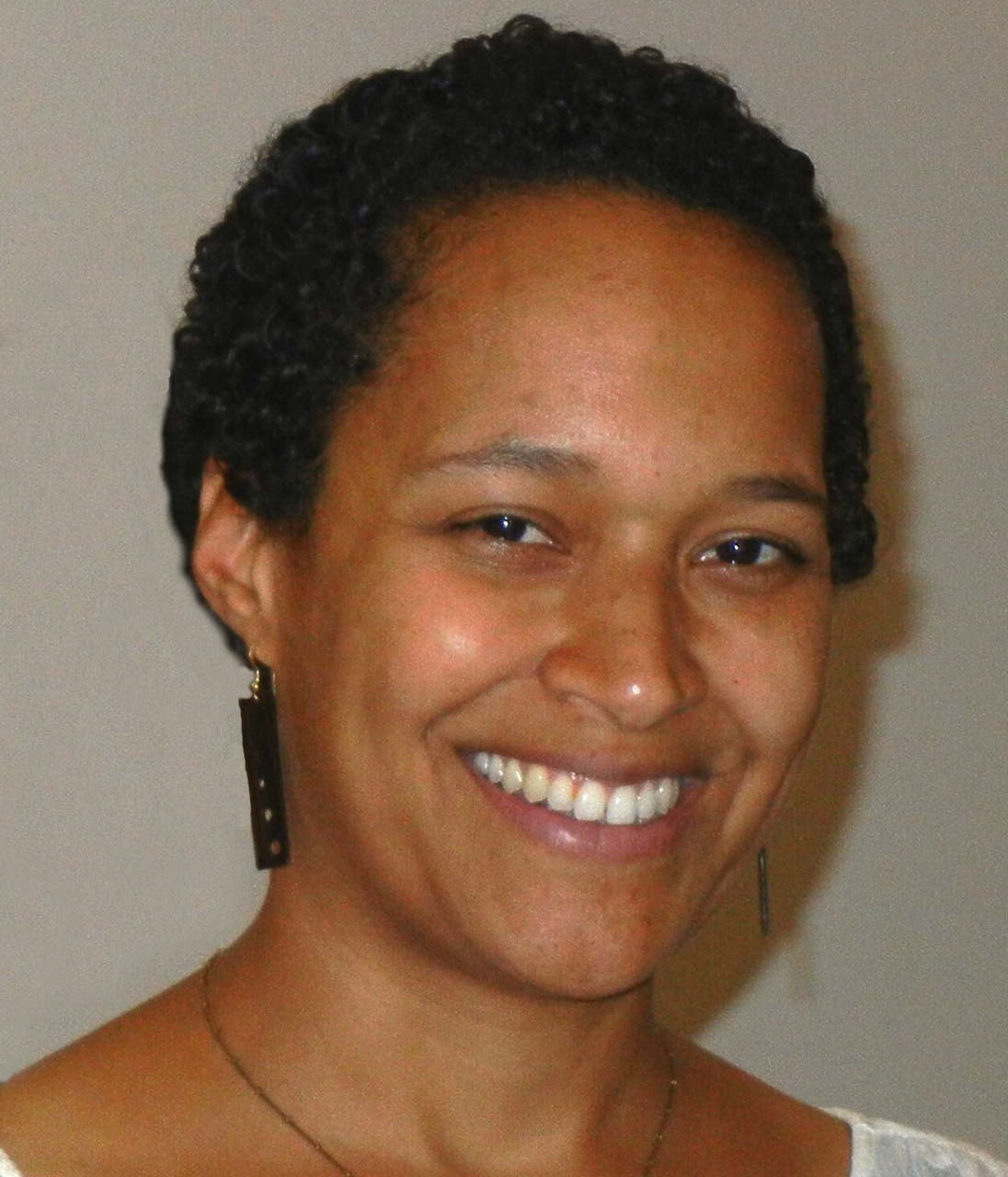
28 Feb From Web to Web: Rebecca Carter SP91 CB92 TA00, Spider Scientist turned Data Scientist
Rebecca Carter has a Ph.D. in Environmental Science, Policy and Management, but she’s eschewed her former work on the behavior and evolution of spiders to delve into data science with the major online auction site eBay. Editor Michael Becker recently sat down with Becca to talk about her unique path to her current career, the fascinating world of data science, and her current service work around supporting people integrating into society following incarceration.
Could you talk a bit about your path to your current position as a data scientist?
Sure! It’s been a bit of a convoluted path. During my undergrad at Cornell, I was a biology person – a nature girl. I was a College Scholar and focused on organismal biology and wildlife studies.As an undergrad, I did research on birds – both field biology and anatomy. Several years after I graduated, I went to UC Berkeley to do a Ph.D where I studied the evolution and behavior of a particular group of spiders on the Hawaiian Islands. However, by around the 4th or 5th year of my doctoral program, I’d realized that I didn’t want to be a professor. By this point, I was no longer super passionate about what I was doing – I would rather just read about it.
About this time, I was serving as President of Telluride Association’s board. It gave me a sense of direction I wasn’t getting at that time from my doctoral program. I had to take a bigger role in figuring out our strategy as an organization, how to handle our endowment, relationships with our university partners – it was really invigorating. It made me want to get back in the workforce.
After I finished my Ph.D, I went into management consulting. I was lucky to find a program through McKinsey that offered a track for disaffected Ph.D.s trying to get a start in the corporate world. I brought to it some basic knowledge from my science training – like statistics – that were useful, but there was a lot else I had to learn on the job. I think TA prepared me well for taking on a new role and figuring it out as I went.
What does your day-to-day work look like?
I do people analytics for the human resources department at eBay. A lot of it involves employing statistical analysis to figure out how to be the best employer we can be.It involves things like looking at the behavior of employees statistically – figuring out factors important to attrition and attraction, looking at what other companies are doing, and applying algorithmic models to HR functions to improve consistency and fairness.
Human resources is often not seen as an analytical place. At eBay, we’re pretty unusual for having 3 data scientists and 4 other data architecture and reporting people in our department. Some days are research – reading new literature, learning new data analysis skills, meeting with people with different human resources specializations. Other days involve analyzing data with SQL/ R/ Python. There’s way too much to do for the time we have, so a lot of it involves figuring out strategically what’s most important and impactful for the company’s vision and mission.
How has TA influenced your path?
TA taught me to have gumption – to have no idea what something was, but to commit to doing it and then having the follow through to get it done. Thanks to my experience with TA, I wasn’t frozen by the feeling of not having the formal business, computer science or mathematical training some of my colleagues did. I’ve had to learn a lot of new things in my current position, but it’s been fun and there’s been a lot of help and support.
In the past few years, there’s been a lot of attention in the news to data science and algorithms – both for the way they’re increasingly supplanting human decision makers, and for some of the sometimes amusing, sometimes disturbing glitches they have. As someone who works heavily in this field, what’s your take?
The general statement the media shares over and over is that if an algorithm does it, it won’t have the same biases people do. But human beings are the ones building algorithms and they’re meant to learn from and mirror data coming from human behavior completely. If you name any stereotype you want, an algorithm will replicate it – and will do what our worst self might imagine. The idea that algorithms are black boxes lets people absolve themselves from the potentially unjust consequences of algorithms’ decisions. For example, there are now algorithms used to determine whether particular prisoners are high risk or not high risk in determining the rights and opportunities they will have in and out of prison. However, the data that these algorithms are based on are things like numbers of previous arrests, report of behavior by guards, etc. – and we know that these factors are influenced by the racial bias already involved in policing, so it ends up amplifying these factors in decision making.
To take another example, biometric measures for things like FitBit and the Apple Watch. Each company had to make a decision about how to read heart rate through the skin of a person’s wrist. FitBit chose to use a green light shone through the skin and measure its absorption into red blood cells; Apple Watch uses an infrared light. Melanin absorbs green light more than infrared light, so on melanated skin, the Apple Watch still reads fairly accurately, but the FitBit fails. It was a choice which method to use, and it was a choice which people to test the hardware on before determining that it worked well enough to go to market. And if a designer or statistician decides to validate their model without attending to a difference like this, you get a product that seems like it works for a high percent of the population, but the people it doesn’t work for are not random, there are pockets of similar people – in this case dark-skinned people – for whom it is much less effective. Designers of hardware and software/algorithms need to critically consider what and who they consider their target population, who they’re testing their product on before it goes to the market. Algorithms capture human behavior, good or bad – designers need to consider more carefully who they’re serving and design their products to be more inclusive.
In addition to your work at eBay, you’re very active in your community. What’s your proudest accomplishment in that realm?
Right now, my husband and I are working on founding a nonprofit that will help support people affected by incarceration. Most people have a natural support network – but it’s often harder for people just coming out of prison. We want to help people rally around that person as they get out of prison and help acclimatize that person to society. We’re trying to find ways to bolster already existing networks, and inject structure and money into those networks when they form.
Loneliness is a big problem for some newly released people – they don’t necessarily have people to turn to when life throws them curveballs – and not having that support can trap people in bad situations. We help form networks of support, both 1:1 guidance and fellowship while people are gaining confidence in their new environment – riding buses, using Google maps to find their way around town, getting a job and housing, figuring out new hobbies, etc – and we develop peer support groups around relationships, money and budgeting. We’re really trying to escape the mold of a lot of the existing service organizations – we don’t operate in the client – service provider binary. We want to help people help themselves, their friends, and their community.
I’ve been really pleased to have the opportunity to make my two worlds intersect. I recently won a Luminary Award from eBay – it’s an award for supporting the cultural values of eBay. I wanted to make sure more people knew about the world around them. I screened Life After Life, a really powerful film about three people’s experiences over several years after their release from San Quentin, looking at what their integration into society looked like. I then brought in several people connected to that experience – a parole lawyer, formerly incarcerated people (including two from the film), people who do job placement for formerly incarcerated people – to talk on a panel about their experiences.
Shara Morris
Posted at 18:53h, 22 MarchHello — I’m a producer with Marketplace’s podcast Make Me Smart with Kai and Molly. I’m interested in getting in touch with Rebecca for a potential interview. Is there a more direct way I could contact her?
Thanks!